Qwen3 Parameter Overview: From 0.6B to 235B, The Ultimate Balance of Hybrid Inference and Multimodality
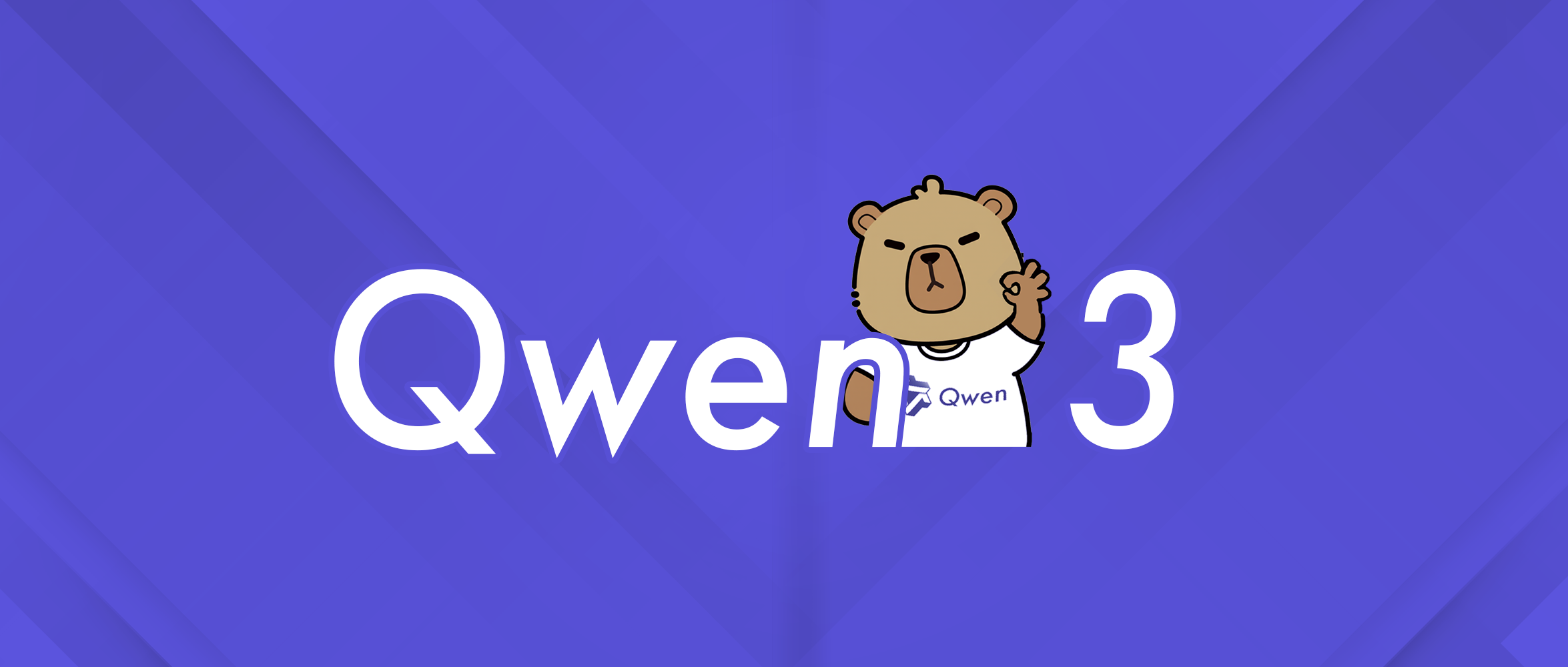
The latest release of the Qwen3 series models by Alibaba Cloud’s Tongyi Qianwen team has drawn industry attention with its diverse model scales and innovative hybrid inference mode. Covering eight models ranging from 0.6B to 235B parameters, Qwen3 not only excels in language, mathematics, and coding tasks but also achieves the ultimate balance between performance and efficiency through MoE (Mixture of Experts) and Dense architectures. The following table details the core parameters and features of the Qwen3 series, revealing its technical core.
Qwen3 Model Parameter Overview
Model Name | Total Parameters | Active Parameters | Architecture Type | Context Length | Supported Languages | License | Key Features |
---|---|---|---|---|---|---|---|
Qwen3-235B-A22B | 235B | 22B | MoE | 128K token | 119 | Qwen License | Flagship model, coding and math capabilities comparable to DeepSeek-R1, Grok-3, efficient inference |
Qwen3-30B-A3B | 30B | 3B | MoE | 128K token | 119 | Qwen License | Small MoE, outperforms Qwen2.5-32B, low inference cost, suitable for local deployment |
Qwen3-32B | 32B | 32B | Dense | 128K token | 119 | Apache 2.0 | High-performance dense model, suitable for complex tasks, inference capability matching Qwen2.5-72B |
Qwen3-14B | 14B | 14B | Dense | 128K token | 119 | Apache 2.0 | Medium scale, balancing performance and resource usage, suitable for enterprise applications |
Qwen3-8B | 8B | 8B | Dense | 128K token | 119 | Apache 2.0 | Lightweight and efficient, suitable for edge devices, performance comparable to Qwen2.5-14B |
Qwen3-4B | 4B | 4B | Dense | 128K token | 119 | Apache 2.0 | Small model, fast inference speed, performance close to Qwen2.5-7B |
Qwen3-1.7B | 1.7B | 1.7B | Dense | 128K token | 119 | Apache 2.0 | Ultra-lightweight, suitable for mobile devices, performance matching Qwen2.5-3B |
Qwen3-0.6B | 0.6B | 0.6B | Dense | 128K token | 119 | Apache 2.0 | Smallest scale, minimal resource requirements, suitable for low-power scenarios |
Recommended Parameter Settings for Local Deployment of Qwen3
Mode | Temperature | TopP | TopK | MinP | Presence Penalty | Ollama Settings | Notes |
---|---|---|---|---|---|---|---|
Thinking Mode | 0.6 Controls randomness, lower values more stable | 0.95 Cumulative probability sampling, higher values increase diversity | 20 Selects top K words, balances diversity | 0 No probability floor, maximum flexibility | 0 ~ 2 Reduces repetition, use high values with caution | num_ctx=40960 num_predict=32768 keep_alive=-1 | Disable greedy decoding to avoid performance degradation and repetition. |
Non-thinking Mode | 0.7 Slightly higher randomness, increases creativity | 0.8 Lower value, more focused output | 20 Selects top K words, balances diversity | 0 No probability floor, maximum flexibility | 0 ~ 2 Reduces repetition, use high values with caution | num_ctx=40960 num_predict=32768 keep_alive=-1 | High presence_penalty may cause language mixing |
Parameter and Feature Analysis
Model Scale and Architecture Type
Qwen3 series offers two architectures:
- MoE (Mixture of Experts): Models like Qwen3-235B-A22B and Qwen3-30B-A3B achieve efficient inference by activating only a portion of parameters (22B or 3B). Despite large total parameter counts, their computational cost is comparable to small-scale dense models. MoE architecture shows excellent performance in coding and mathematical tasks with significantly improved inference speed.
- Dense (Dense Model): Full-parameter models ranging from 0.6B to 32B, suitable for scenarios requiring stable high performance. Small models (like Qwen3-0.6B) are optimized for edge devices, while large models (like Qwen3-32B) excel in complex inference tasks.
Context Length
All Qwen3 models support a context length of 128K tokens, capable of handling extra-long documents or multi-turn dialogues, with generation capability up to 8K tokens. This feature provides a clear advantage in long-text generation and document comprehension tasks.
Multilingual Support
Qwen3 supports 119 languages and dialects, covering Chinese, English, European languages, and low-resource languages, making it suitable for global multilingual application scenarios.
Hybrid Thinking Mode
Qwen3 pioneers the switching between thinking and non-thinking modes:
- Thinking Mode: Uses chain-of-thought (CoT) reasoning for step-by-step deduction, suitable for complex mathematical, coding, and logical reasoning tasks.
- Non-thinking Mode: Provides quick responses to simple queries, optimizing latency and computational cost.
This design is achieved through four-stage training (long CoT cold start, reasoning-based RL, thinking mode fusion, general RL), significantly improving task adaptability.
License and Open Source Strategy
- Dense Models (0.6B-32B) use the Apache 2.0 license, suitable for commercial applications.
- MoE Models (235B-A22B, 30B-A3B) use the Qwen License, more suitable for research scenarios.
Performance and Efficiency
Benchmark Performance
- Qwen3-235B-A22B: Competes with top models like DeepSeek-R1 and Grok-3 in tests such as MMLU-Pro and LiveCodeBench, with particularly outstanding coding and mathematical capabilities.
- Qwen3-30B-A3B: Despite activating only 3B parameters, it surpasses Qwen2.5-32B with 10x improvement in inference efficiency, suitable for local deployment and real-time applications.
- Small Models: Models like Qwen3-4B perform comparably to Qwen2.5-72B, suitable for resource-constrained scenarios.
Related Links
More Articles
![OpenAI 12-Day Technical Livestream Highlights Detailed Report [December 2024]](/_astro/openai-12day.C2KzT-7l_1ndTgg.jpg)
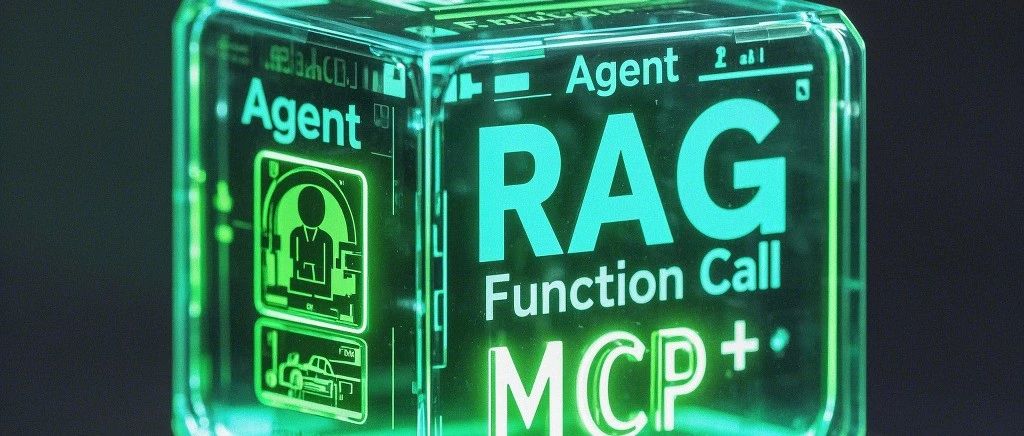
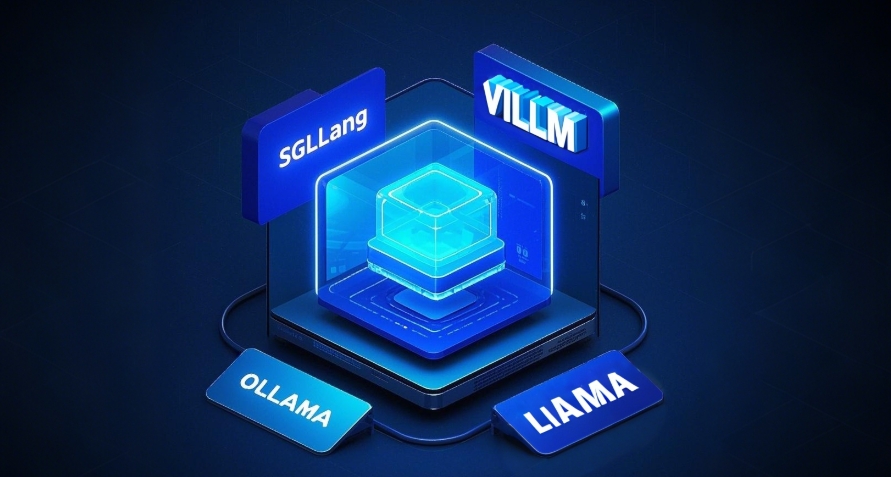
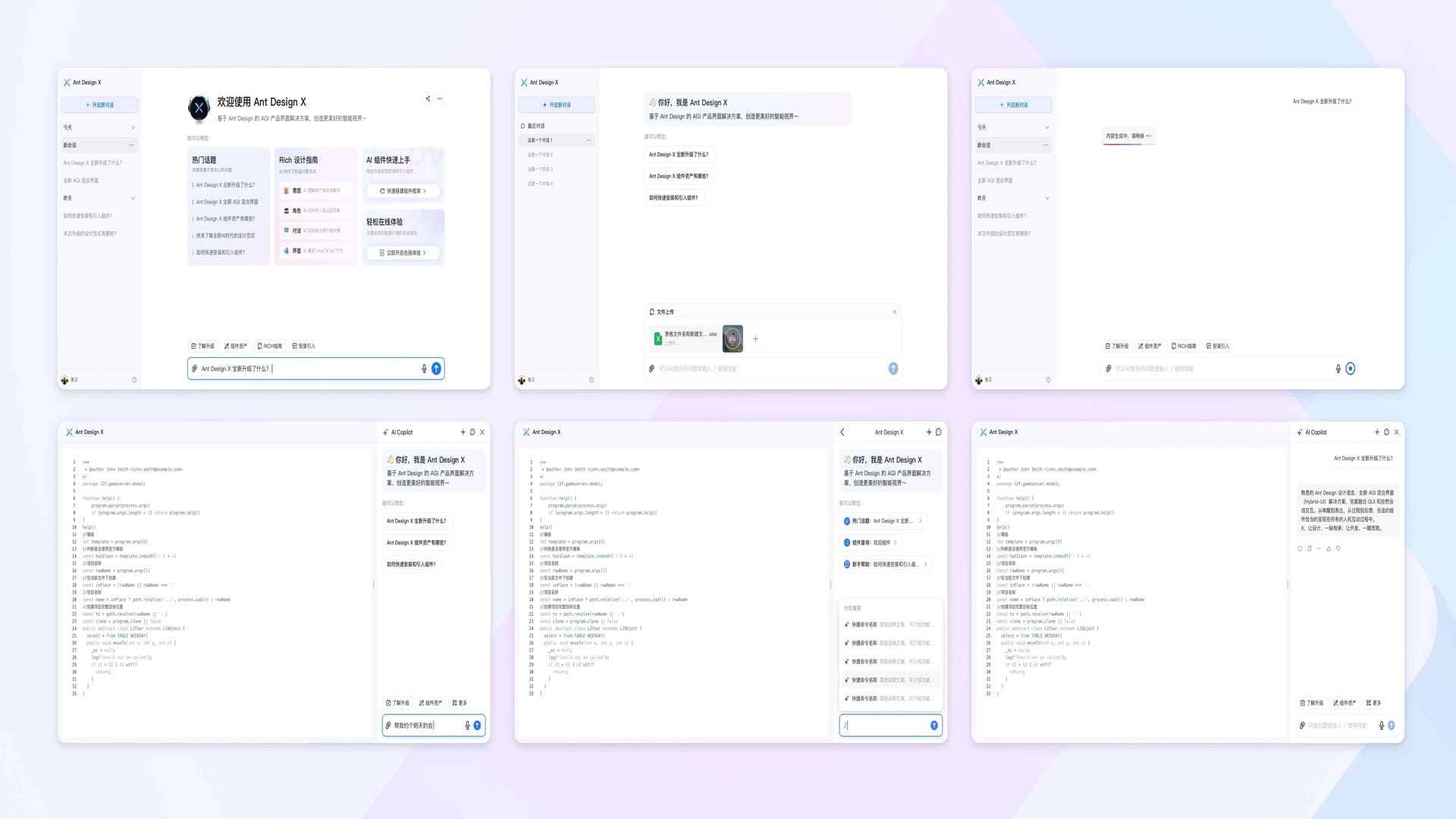
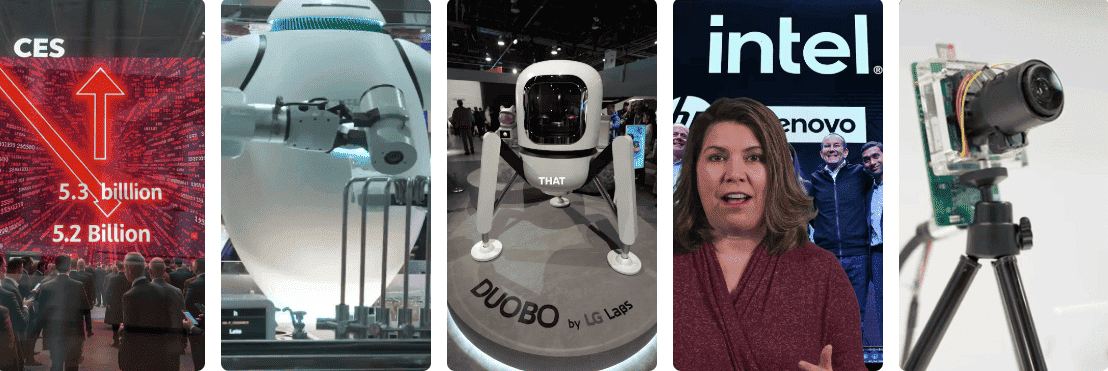
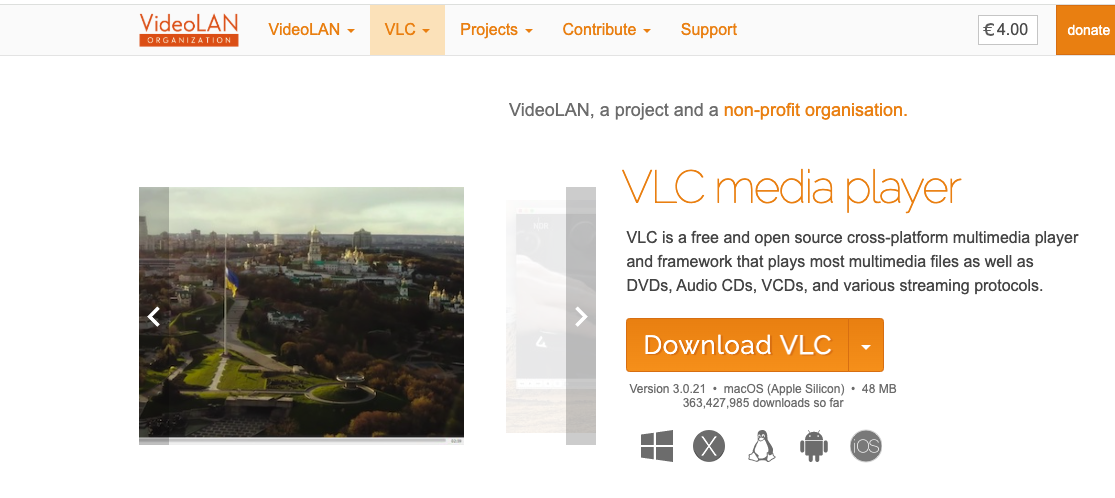
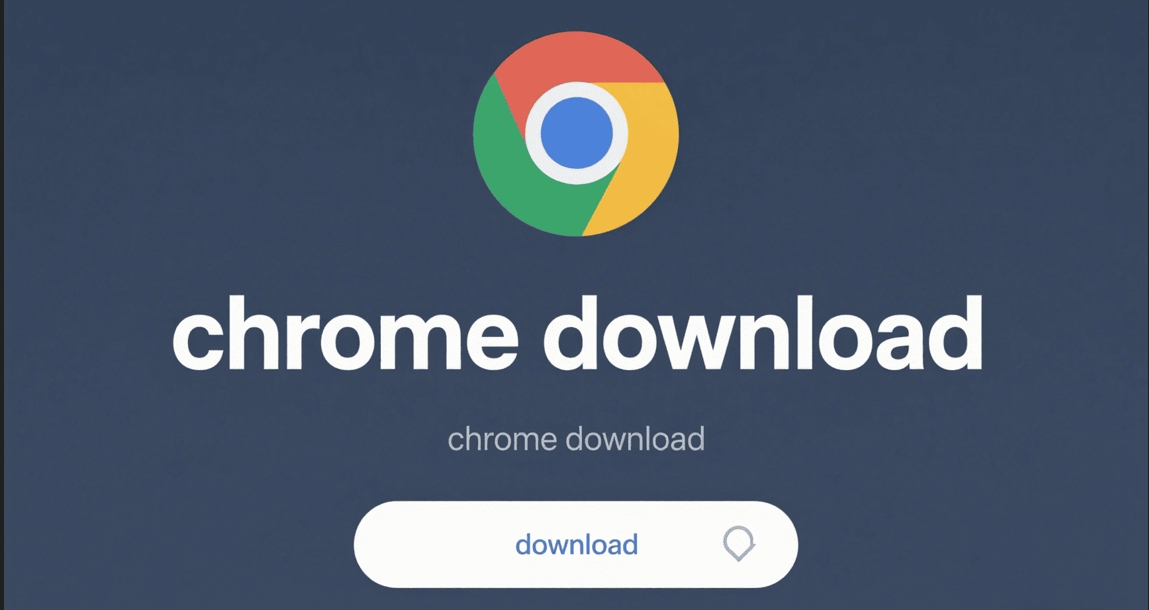
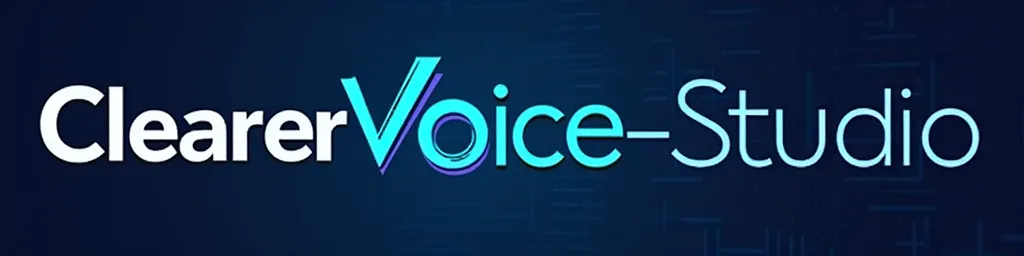
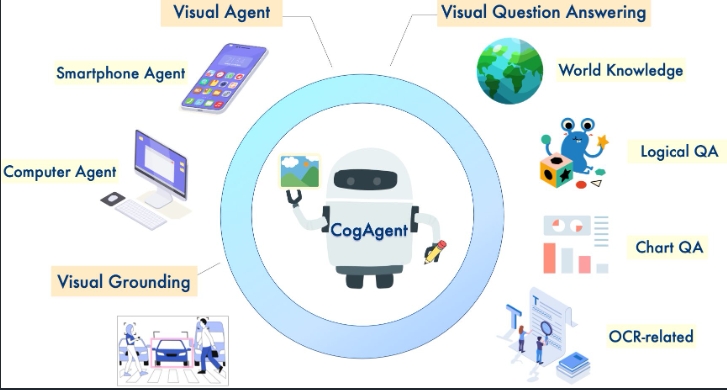