Qwen2.5-Omni Flagship vs MiniCPM-V Powerhouse: Complete Analysis of Parameters, Hardware, Resources, and Advantages
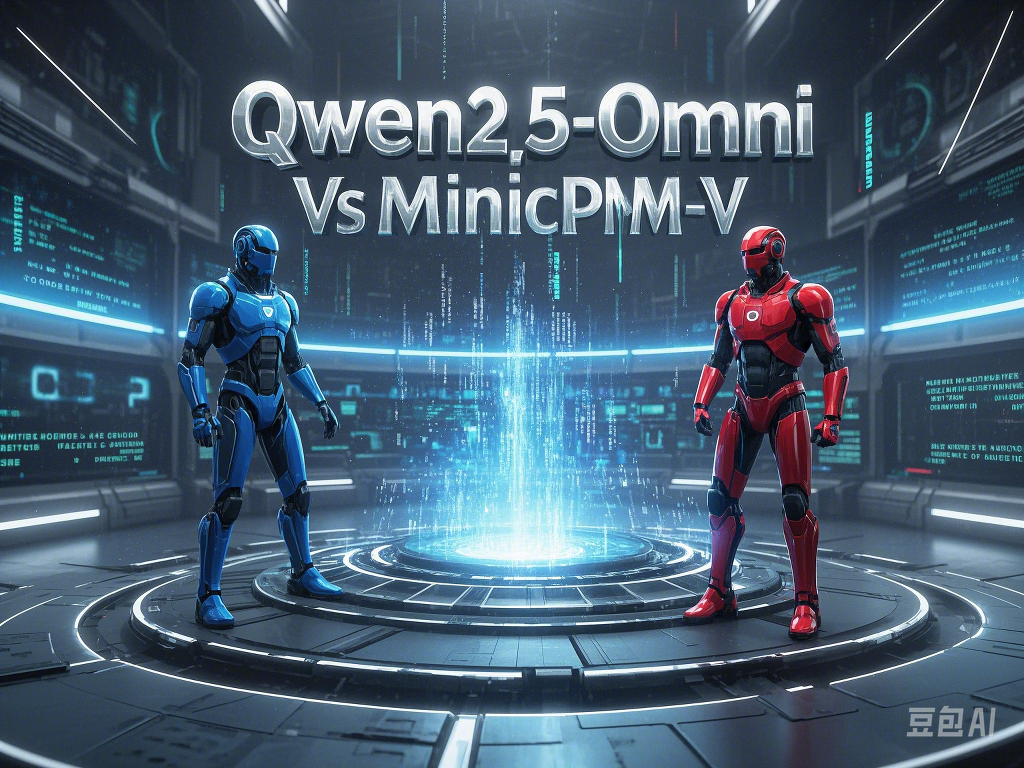
Recently, Alibaba’s Qwen2.5-Omni
has been gaining significant attention. It can hear, speak, see, and think, with real-time processing capabilities, embodying the future of human-machine interaction. Speaking of trending models, there’s also the “compact powerhouse” called MiniCPM-V
, developed with participation from Tsinghua University. What makes it remarkable is its ability to run on mobile phones while delivering exceptional visual understanding capabilities, truly bringing AI “large model” technology to edge deployment. This article analyzes the technical details and deployment conditions of these two models, as well as their future development directions.
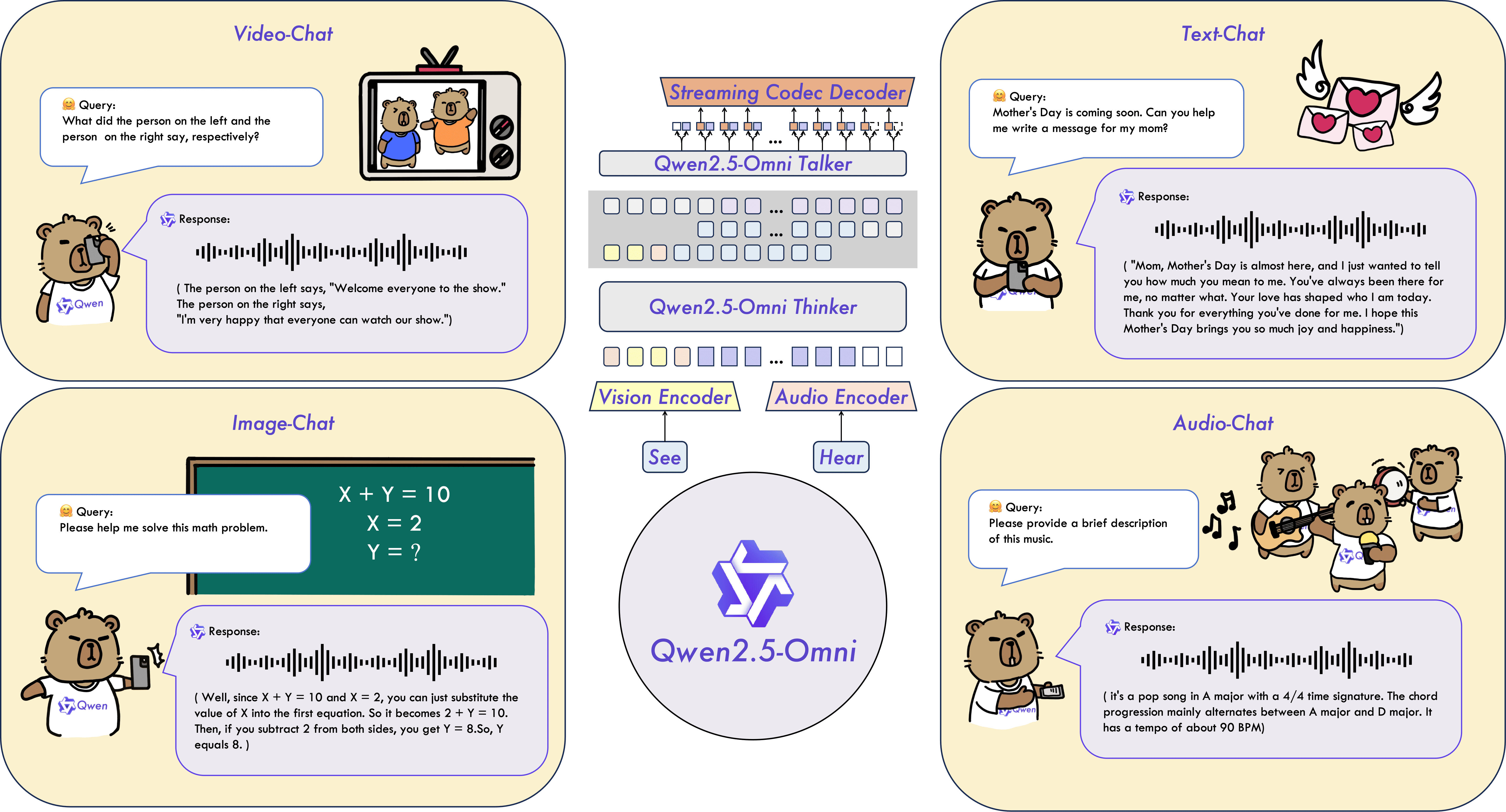
I. Architectural Philosophy and Core Positioning: Comprehensive Exploration vs. Efficiency Specialization
- Qwen2.5-Omni: Its core is the Thinker-Talker architecture, designed to build a unified end-to-end model. The Thinker (based on Qwen2.5 LLM) is responsible for deep understanding and reasoning of cross-modal information, generating text responses; while the Talker, using the Thinker’s hidden representations and text output, specializes in real-time streaming generation of high-quality speech. Meanwhile, the TMRoPE positional encoding solves the critical problem of audio-video temporal alignment. Its positioning is to become a universal multimodal agent with extensive perception and expression capabilities that closely resembles human interaction modes.
- Source: (Qwen2.5-Omni Paper)
- MiniCPM-V: Adopts a combination of efficient visual encoder (SigLIP) + lightweight language model (MiniCPM series). Its core design philosophy is to achieve the best balance between performance and efficiency, with particular focus on application potential in resource-constrained edge devices. Its V2.0 version introduces Adaptive Visual Encoding technology, supporting the processing of high-resolution images with arbitrary aspect ratios, significantly enhancing performance on fine-grained visual tasks such as OCR. Its positioning is to become an efficient, reliable, and easily deployable vision-language model.
- Source: MiniCPM-V Paper.
II. Model Scale and Resource Requirements: Heavyweight vs. Lightweight
- Qwen2.5-Omni (7B):
- Scale: 7 billion parameters, a medium-large model.
- Resource requirements (full precision): Requires tens of GB of GPU memory, primarily targeting cloud or high-performance computing environments.
- Resource requirements (quantized): Through INT4 and other quantization techniques, it can run on consumer-grade GPUs with 12GB+ VRAM, but additional attention must be paid to the overhead and adaptation issues brought by multimodal components.
- MiniCPM-V (2.8B):
- Scale: Approximately 2.8 billion parameters, significantly smaller than Qwen7B.
- Resource requirements (quantized): After INT4 quantization, the model memory usage is only about 5GB. With compilation and memory optimization, it can run smoothly on mainstream smartphones and other devices with extremely limited memory. It has low inference computational requirements and high energy efficiency.
The positioning difference between the two in terms of scale and resource requirements is evident. Qwen tends toward cloud high-performance computing, while MiniCPM-V takes edge low-resource deployment as its core objective and has undergone deep optimization for this purpose.
III. Deployment Conditions and Optimization Practices: Cloud-First vs. Edge-Benchmark
- Qwen2.5-Omni: Primarily relies on cloud GPU deployment to achieve full performance. The feasibility of local deployment depends on quantized models provided by the community and users’ higher-specification consumer-grade graphics cards.
- MiniCPM-V: Edge deployment is its core competitive advantage. A comprehensive set of optimization strategies including 4-bit quantization, time-division memory loading (ViT/LLM), compilation optimization, CPU configuration auto-search, NPU acceleration enable MiniCPM-V to achieve decoding throughput (8.2 tokens/s) on mainstream flagship phones that matches or even exceeds human reading speed, while significantly reducing visual encoding latency.
MiniCPM-V not only has high feasibility in edge deployment but has also formed a mature set of optimization methodologies and practical cases, Android and other edge deployment official examples. The localization potential of Qwen2.5-Omni depends more on third-party toolchains and community contributions.
IV. Core Capabilities and Comparative Advantages: Interaction Dimensions vs. Visual Efficiency
-
Qwen2.5-Omni (7B):
- Advantages:
- Multimodal breadth: Audio, video input, and real-time streaming speech output are its unique advantages, building a more complete interaction capability.
- Mixed modal fusion: Leading capability in tasks requiring simultaneous understanding of information from multiple sources (such as OmniBench).
- Voice instruction understanding: High level of response to natural voice instructions, approaching text input.
- Limitations (relative): High threshold for edge deployment, strict resource requirements.
- Advantages:
-
MiniCPM-V (2.8B):
- Advantages:
- Efficient visual understanding: Achieves top-tier VLM performance with a small model size, especially outstanding in OCR (supporting high-resolution images with arbitrary aspect ratios).
- Excellent deployment efficiency: Low resource usage, high inference speed, easy integration into mobile and edge applications.
- Reliability: Effectively reduces content hallucinations through technologies such as RLAIF-V.
- Multilingual capability: Can effectively extend to image-text understanding in more than 30 languages.
- Limitations (relative): Does not natively support audio, video input, and speech output, but there is a dedicated speech model MiniCPM-o.
- A note on MiniCPM-O speech model performance:
MiniCPM-o 2.6 scores an average of 70.2 on OpenCompass, a comprehensive evaluation of 8 popular benchmarks. With just 8B parameters, it surpasses widely-used proprietary models like GPT-4o-202405, Gemini 1.5 Pro, and Claude 3.5 Sonnet in single-image understanding. It also outperforms GPT-4V and Claude 3.5 Sonnet in multi-image and video understanding, and demonstrates good in-context learning ability.
- Advantages:
V. Selection Considerations and Future Outlook
Key Consideration | Favoring Qwen2.5-Omni (7B) | Favoring MiniCPM-V (2.8B) |
---|---|---|
Core Application Scenarios | Complex systems requiring audio-video understanding and real-time voice interaction | Image/document understanding, OCR, edge/mobile visual applications |
Deployment Environment | Cloud / High-performance GPU / High-spec consumer GPU | Mobile devices / Edge computing / Regular PC |
Resource/Cost Sensitivity | Low | High |
Interaction Modal Requirements | Requires hearing, speaking, seeing full chain | Core is seeing (image/text) |
Development Focus | Exploring cutting-edge full-modal interaction experiences | Quick landing, efficiency priority, edge intelligence |
Conclusion:
Qwen2.5-Omni
and MiniCPM-V
represent two important paradigms in the evolution of multimodal AI. Qwen2.5-Omni
, with its comprehensive modal coverage and real-time speech capabilities, pushes multimodal interaction to new heights and is more suitable for building powerful intelligent applications in the cloud. Meanwhile, MiniCPM-V
, through extreme optimization, proves that achieving high-performance, low-cost, reliable visual intelligence on edge devices is entirely feasible. We believe that with the subsequent development of AI, even smaller and more powerful models will continue to emerge.
More Articles
![OpenAI 12-Day Technical Livestream Highlights Detailed Report [December 2024]](/_astro/openai-12day.C2KzT-7l_1ndTgg.jpg)
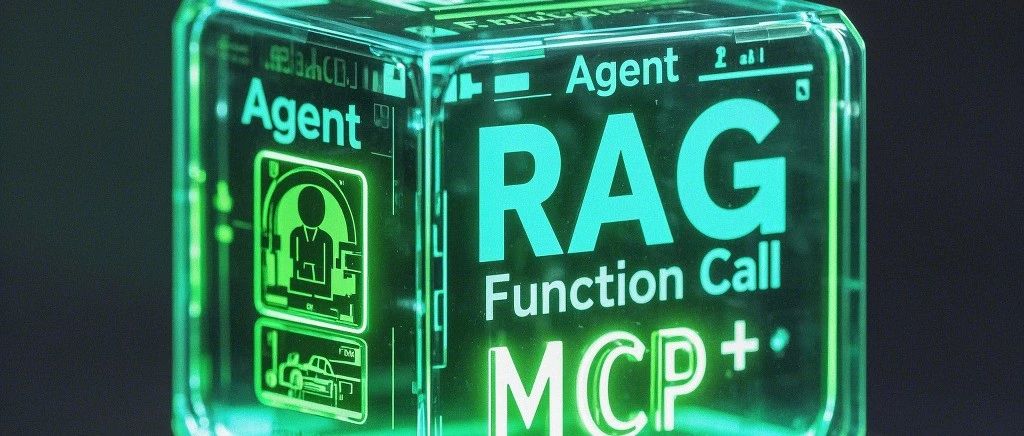
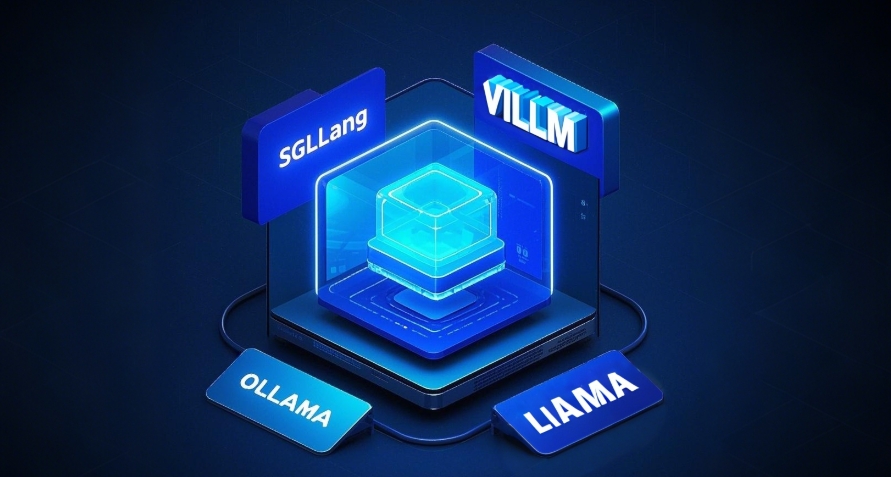
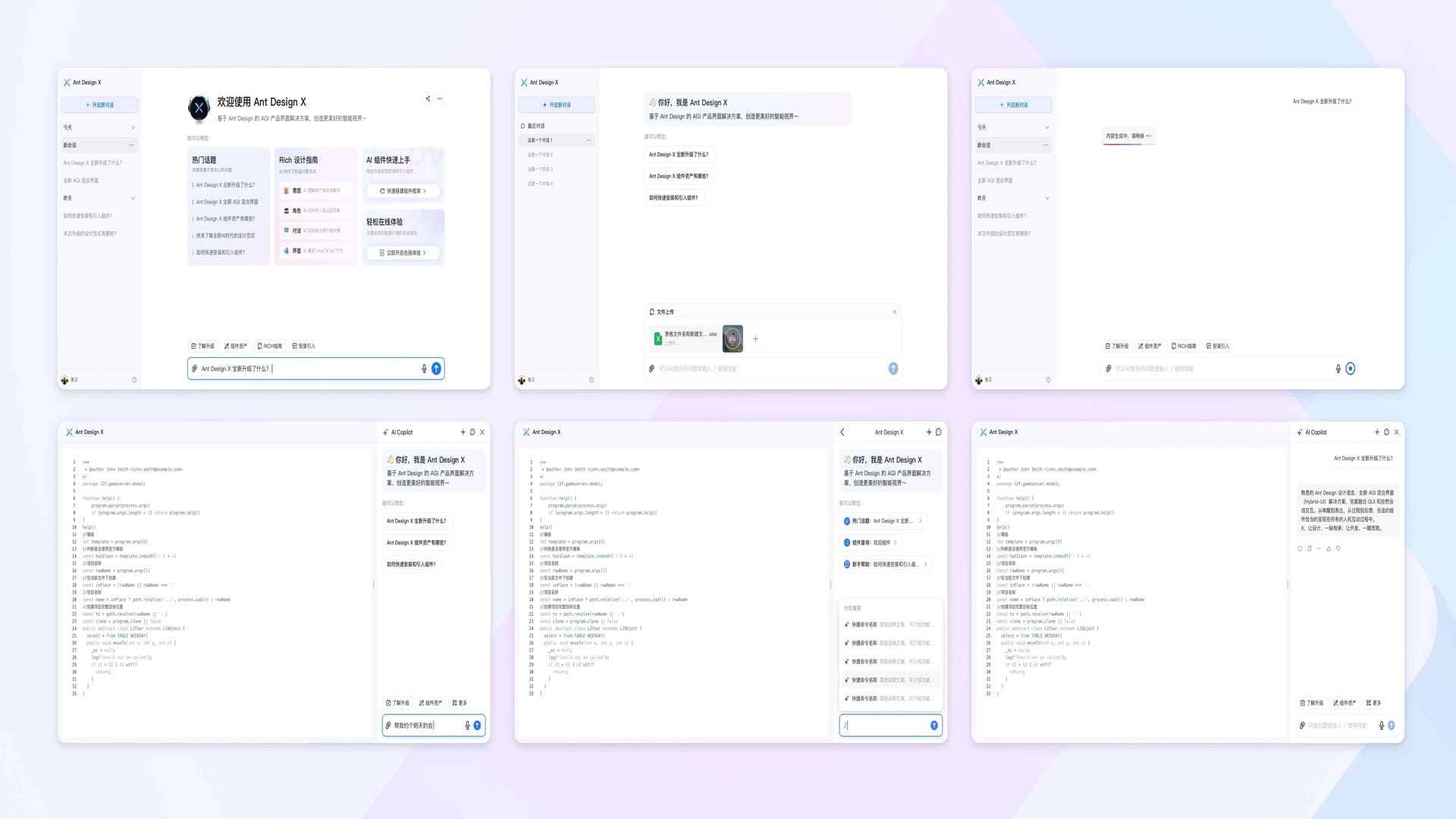
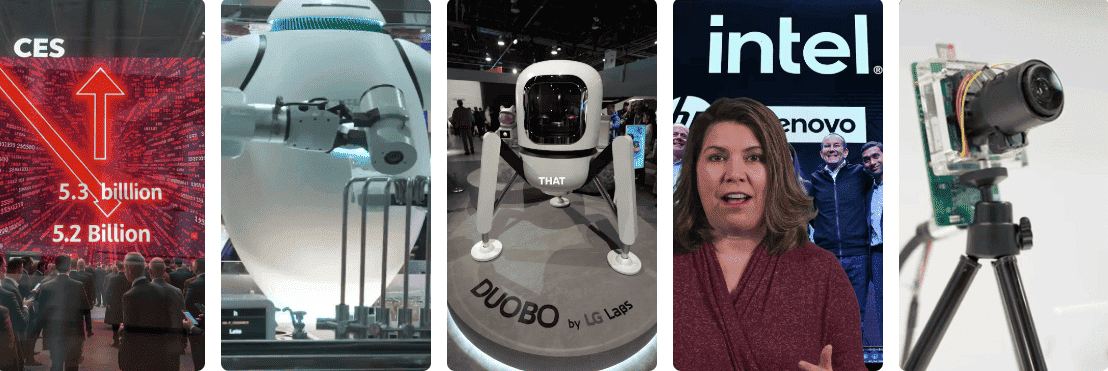
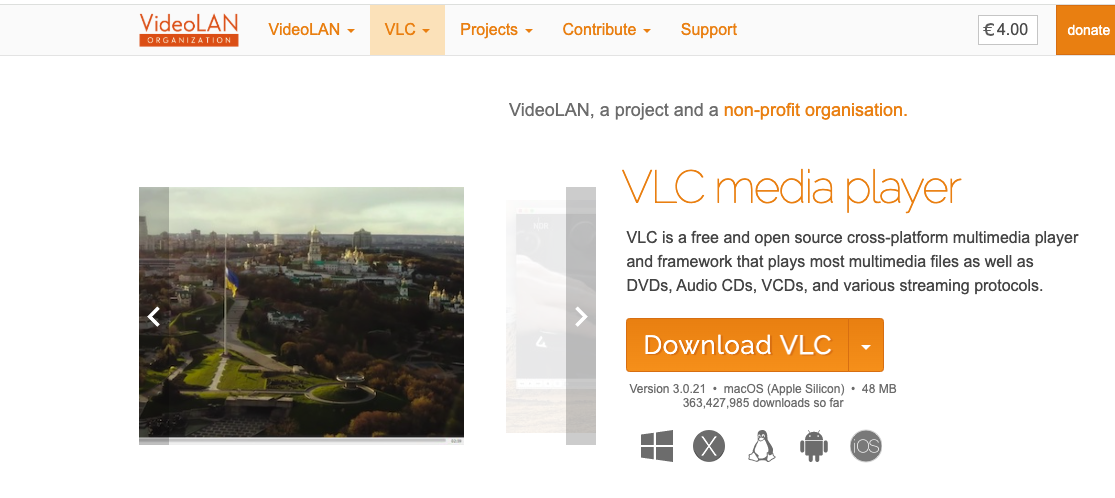
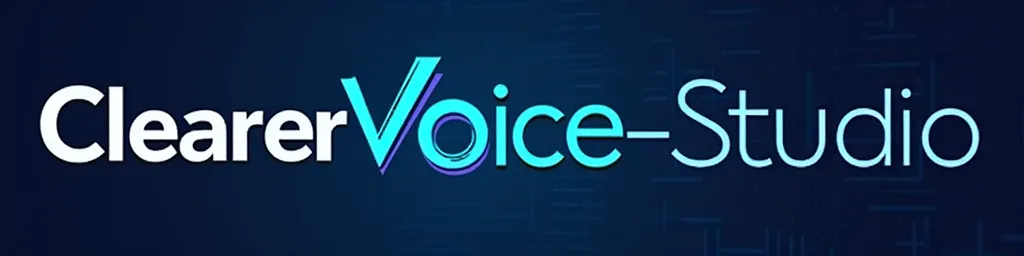
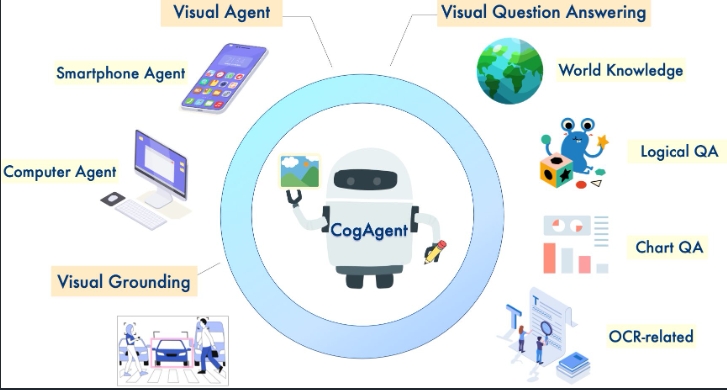
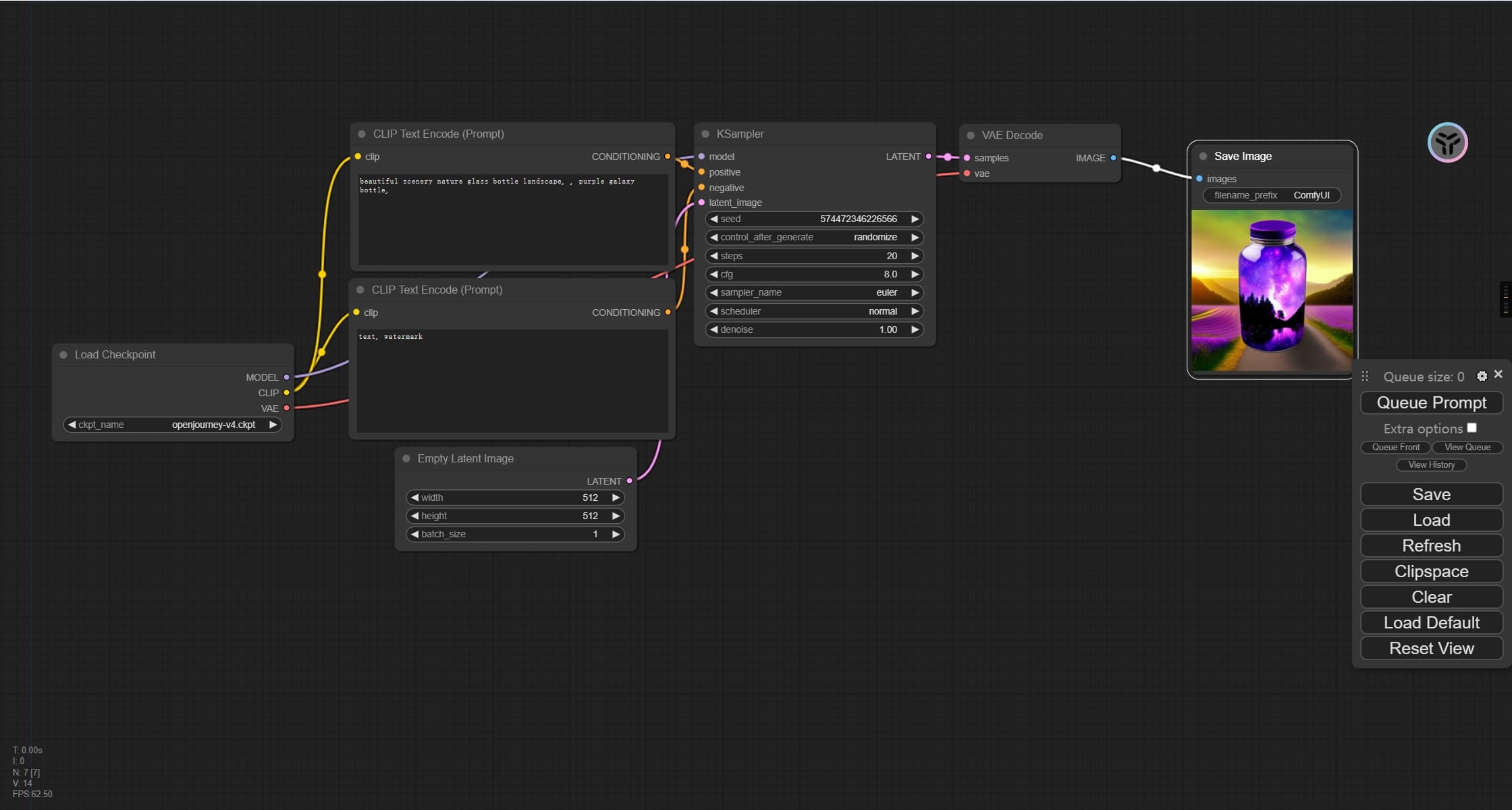