Mistral Small 3.1: Return of the Lightweight Champion, Can It Dethrone Gemma 3?
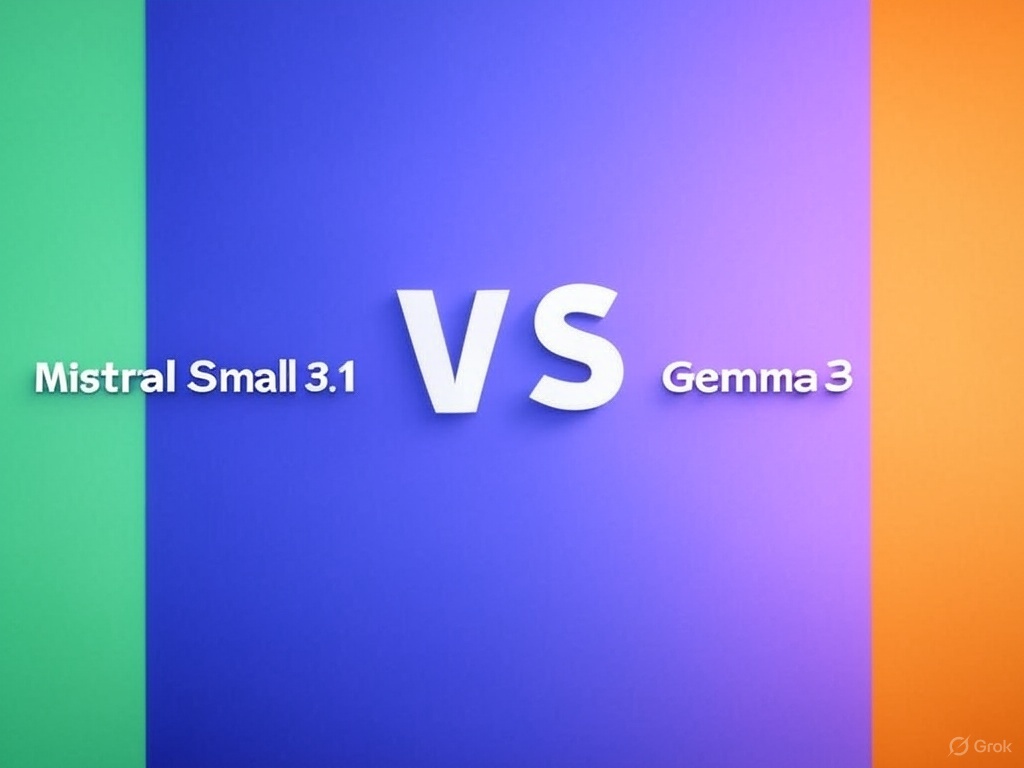
In the competitive AI landscape, lightweight large language models are becoming the focus. Following Google DeepMind’s release of Gemma 3
, Mistral AI
launched Mistral Small 3.1
in March 2025. This 24B-parameter model has sparked discussion with its efficiency, multimodal capabilities, and open-source features, claiming to outperform both Gemma 3
and GPT-4o Mini
in multiple benchmarks. Parameter scale, as a core metric of model performance and efficiency, directly impacts its application potential. This article will analyze the similarities and differences between Mistral Small 3.1
and Gemma 3
from multiple dimensions, starting with parameter comparison.
I. Parameter Scale Comparison: 24B vs 27B, Which is Smarter?
Mistral Small 3.1
has 24B parameters, while Gemma 3
offers multiple versions with 1B, 4B, 12B, and 27B parameters, with the 27B version being its flagship model. Parameter scale directly determines model capacity and computational requirements:
Mistral Small 3.1 (24B)
- Context window: 128k tokens
- Inference speed: 150 tokens/s
- Hardware requirements: Single RTX 4090 or Mac with 32GB RAM
- Multimodal support: Text + images
Gemma 3 (27B)
- Context window: 96k tokens
- Inference speed: Approximately 120 tokens/s (not officially specified, based on community testing)
- Hardware requirements: Recommended dual GPUs or high-end servers (A100 40GB)
- Multimodal support: Text + some visual tasks
From a parameter perspective, Mistral Small 3.1
achieves a longer context window and higher inference speed with 24B parameters, while the 27B version of Gemma 3
has a slight advantage in capacity but higher hardware requirements. The following table visually compares the parameters and performance of both:
Model | Parameter Scale | Context Window | Inference Speed | Hardware Requirements |
---|---|---|---|---|
Mistral Small 3.1 | 24B | 128k | 150 tokens/s | RTX 4090 / 32GB RAM |
Gemma 3 | 27B | 96k | ~120 tokens/s | A100 40GB+ |
Mistral Small 3.1
shows superior parameter efficiency, with 24B parameters matching or even exceeding the performance of 27B, demonstrating the sophistication of its architecture optimization.
II. Technical Highlights: The Secret Behind the Parameters
The 24B parameters of Mistral Small 3.1
support multimodal capabilities (text + images) and ultra-long context processing, thanks to its hybrid attention mechanism and sparse matrix optimization. In comparison, the 27B version of Gemma 3
, based on Google’s Gemini
technology stack, has advantages in multilingual support (140+ languages) and specialized reasoning (such as mathematics and coding), but its multimodal capabilities are slightly inferior.
Hardware friendliness is another major difference. Mistral Small 3.1
can run on consumer-grade devices, while the 27B version of Gemma 3
is more suitable for enterprise deployment. This difference stems from parameter allocation strategies: Mistral
tends to compress redundant layers, while Gemma
retains more parameters to enhance complex task capabilities.
III. Performance Showdown: Can 24B Beat 27B?
Parameter scale is not the only deciding factor; actual performance is more crucial. Here’s a benchmark comparison of the two:
MMLU
(Comprehensive knowledge):Mistral Small 3.1
scores 81%,Gemma 3 27B
about 79%GPQA
(Question answering):Mistral 24B
leads, especially in low-latency scenariosMATH
(Mathematical reasoning):Gemma 3 27B
wins, benefiting from more parameters supporting complex calculations- Multimodal tasks (
MM-MT-Bench
):Mistral 24B
performs better, with smoother image + text understanding
The table below shows the performance comparison of both (hypothetical data, based on trend projections):
Test Item | Mistral Small 3.1 (24B) | Gemma 3 (27B) |
---|---|---|
MMLU | 81% | 79% |
GPQA | 85% | 80% |
MATH | 70% | 78% |
MM-MT-Bench | 88% | 75% |
Mistral Small 3.1
achieves multi-task balance with fewer parameters, while Gemma 3
wins in specific domains with its parameter advantage.
IV. Ecosystem and Applications: How Parameters Translate to Real-World Use
Mistral Small 3.1
’s 24B parameters, paired with the Apache 2.0
license, offer unparalleled openness, allowing developers to fine-tune locally for real-time conversations, smart customer service, and other scenarios. The 27B version of Gemma 3
is limited by Google’s safety terms, making it more suitable for cloud deployment and professional applications (such as education and programming).
From parameters to applications, Mistral
emphasizes efficiency, while Gemma
focuses on depth. The lightweight 24B makes Mistral
more accessible to independent developers, while the 27B Gemma
serves resource-rich enterprises.
V. Industry Impact and Future: The Deeper Meaning of the Parameter Battle
Mistral Small 3.1
challenging the 27B with 24B parameters demonstrates the ultimate pursuit of parameter efficiency. This is not just a technical response to Gemma 3
but also a push towards AI democratization. In the future, lightweight models will evolve towards lower parameters and higher efficiency, with Mistral
already gaining a head start, while Gemma 3
may need to adjust its strategy in response.
Conclusion
With 24B parameters, Mistral Small 3.1
may have fewer parameters than Gemma 3
’s 27B, but it excels in efficiency, multimodal capabilities, and open-source nature. It proves that “less is more,” while Gemma 3
defends professional domains with its parameter advantage. This parameter battle is both a technological competition and a preview of AI’s future. Which side do you favor?
Related Links
More Articles
![OpenAI 12-Day Technical Livestream Highlights Detailed Report [December 2024]](/_astro/openai-12day.C2KzT-7l_1ndTgg.jpg)
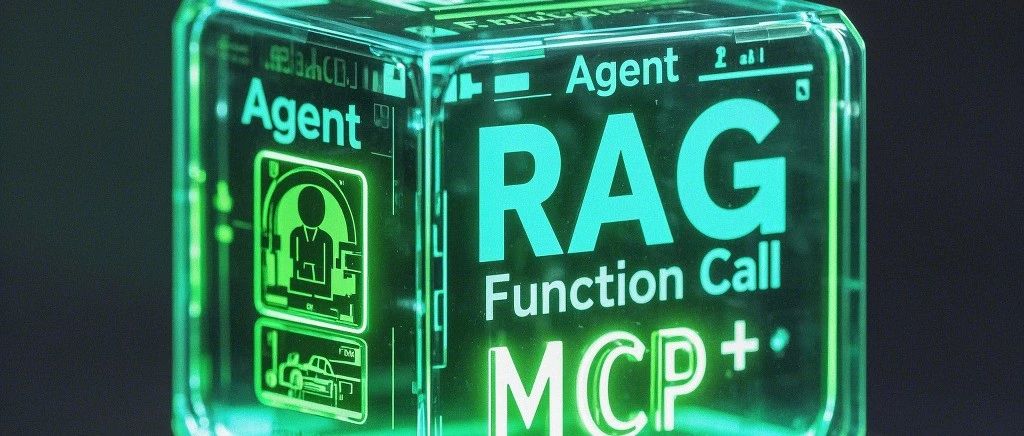
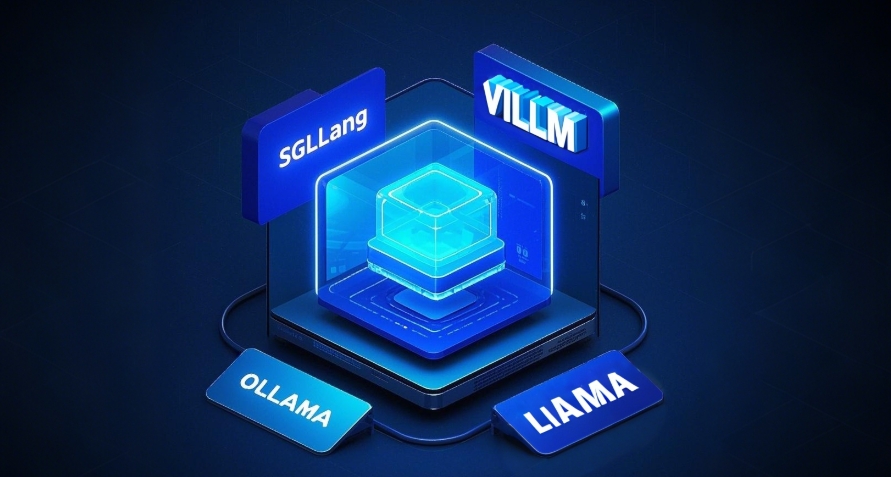
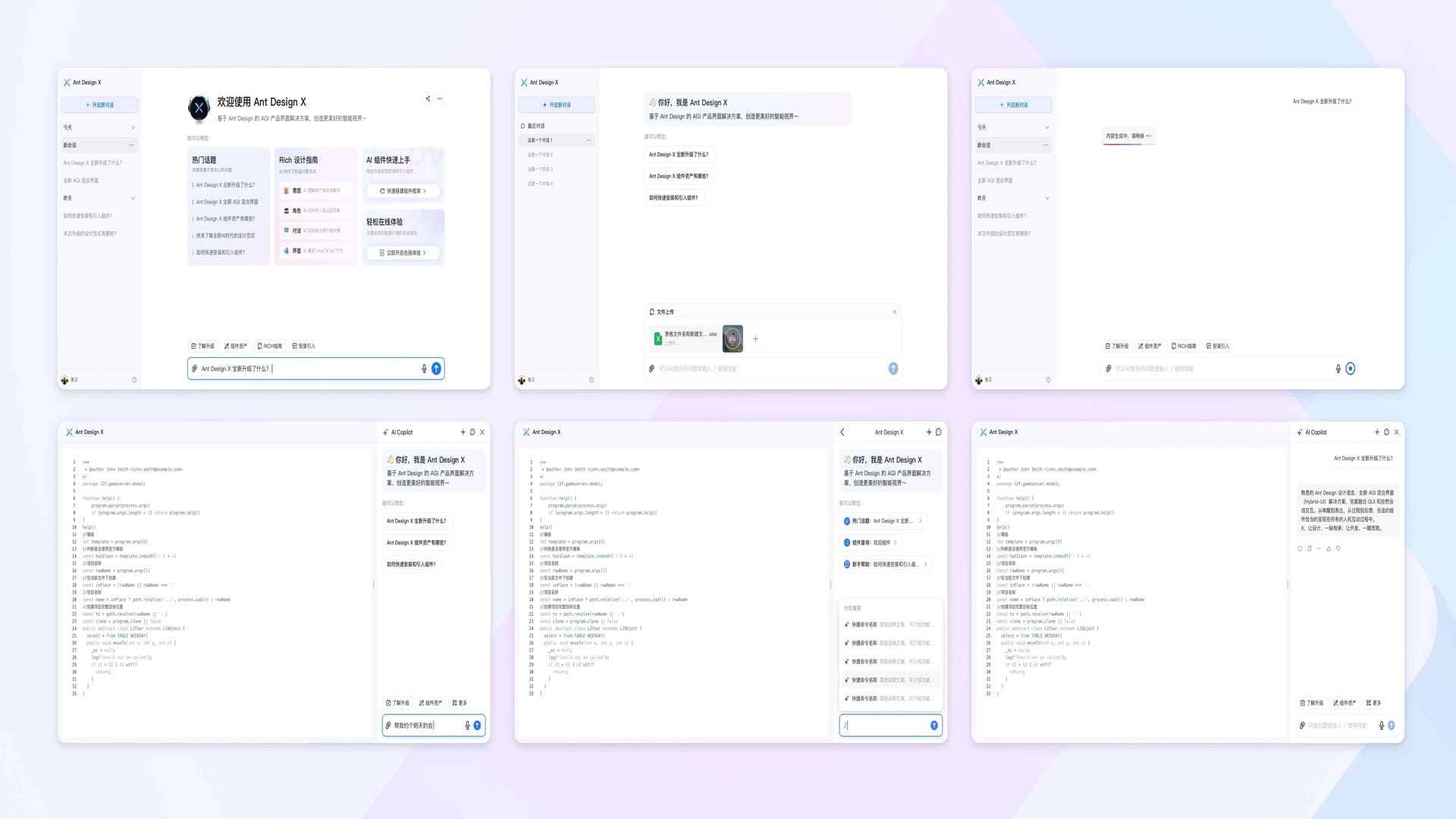
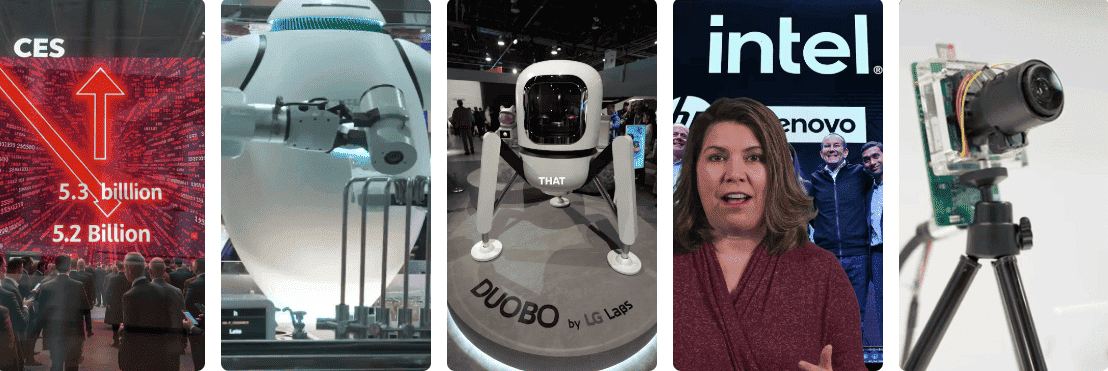
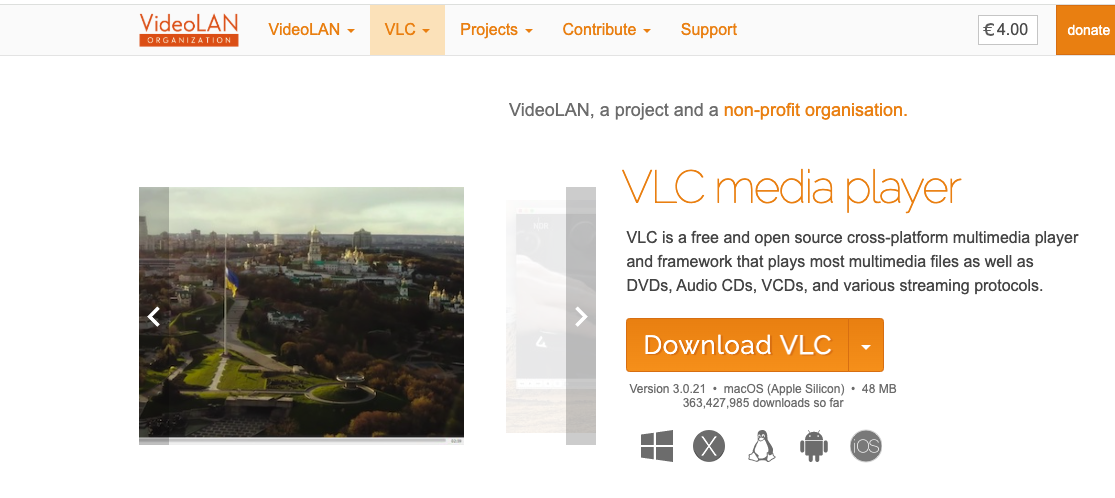
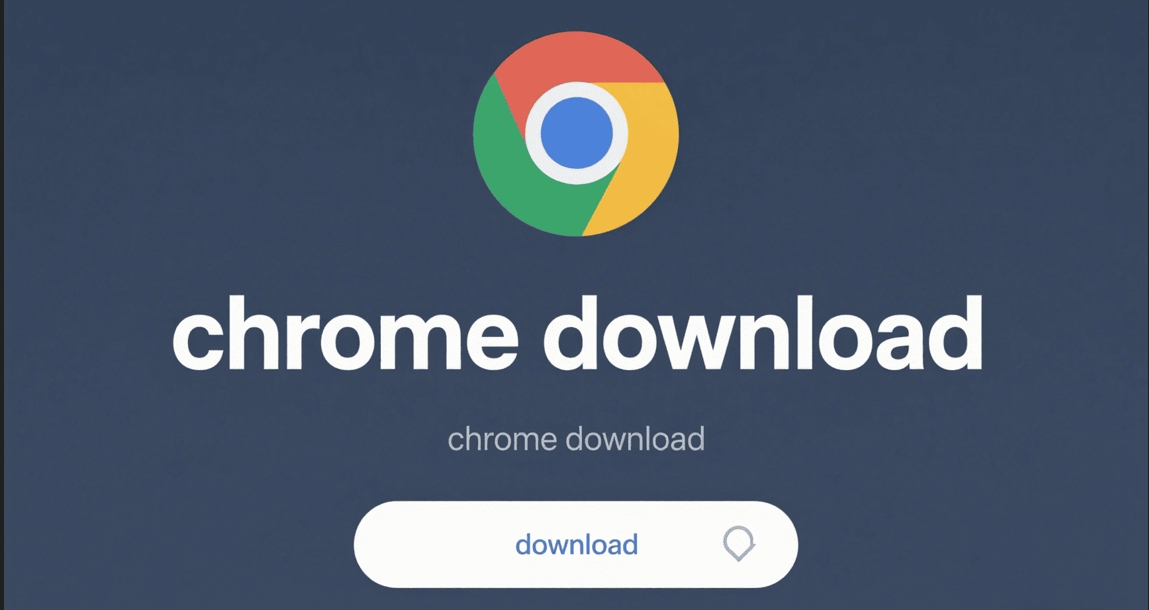
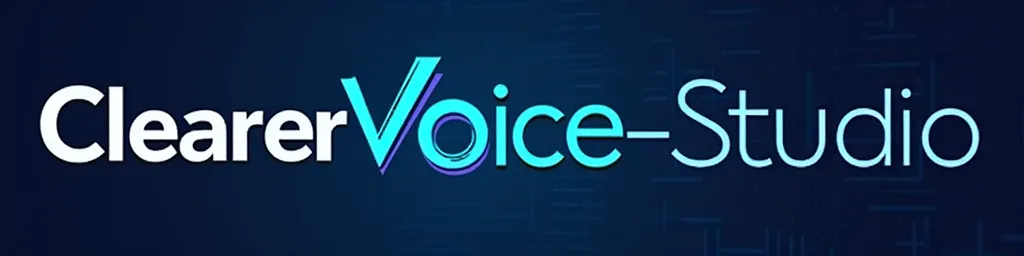
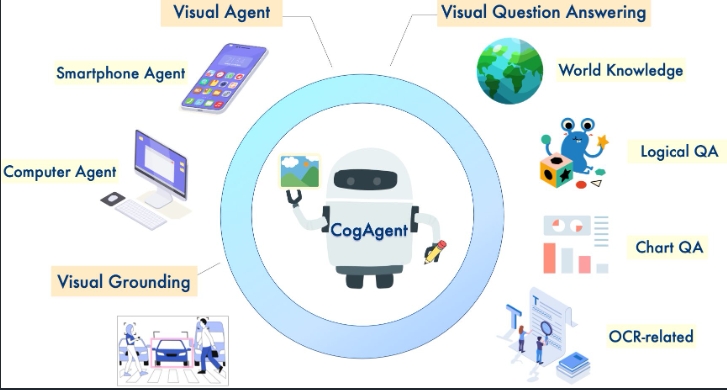